ML | Gaussian-Process (GP)
In probability theory and statistics, a Gaussian process is a stochastic process (a collection of random variables indexed by time or space), such that every finite collection of those random variables has a multivariate normal distribution. The distribution of a Gaussian process is the joint distribution of all those (infinitely many) random variables, and as such, it is a distribution over functions with a continuous domain, e.g. time or space.
From the above derivation, you can view Gaussian process as a generalization of multivariate Gaussian distribution to infinitely many variables. Here we also provide the textbook definition of GP, in case you had to testify under oath:
A Gaussian process is a collection of random variables, any finite number of which have consistent Gaussian distributions.
\[ f(x) \sim \mathcal{G}\mathcal{P}(m(x), K(x, x')) \]
A Gaussian process is a probability distribution over possible functions that fit a set of points.
Just like a Gaussian distribution is specified by its mean and variance, a Gaussian process is completely defined by
- a mean function \(m(x)\) telling you the mean at any point of the input space and
- a covariance function \(K(x,x')\) that sets the covariance between points.
The mean can be any value and the covariance matrix should be positive definite.
The GP approach, in contrast, is a non-parametric approach, in that it finds a distribution over the possible functions \(f(x)\) that are consistent with the observed data. As with all Bayesian methods it begins with a prior distribution and updates this as data points are observed, producing the posterior distribution over functions.
\[ \begin{align} p(\mathbf{y} | \Sigma ) \approx \exp (-\frac{1}{2} \mathbf{y}^T \Sigma \mathbf{y}) \end{align} \]
\[ \Sigma (x_1, x_2) = K(x_1, x_2) + I \sigma_y^2 \]
The covariance matrices in all the above examples are computed using the Radial Basis Function (RBF) kernel and \(\sigma_y\) can be noise term.
- Here is an example of a 2D Gaussian distribution with mean 0, with the oval contours denoting points of constant probability.
- we can now visualize the sampling operation in a new way by taking multiple "random points"
- For conditioning, we can simply fix one of the endpoint on the index graph (in below plots, fix y1 to 1) and sample from y2
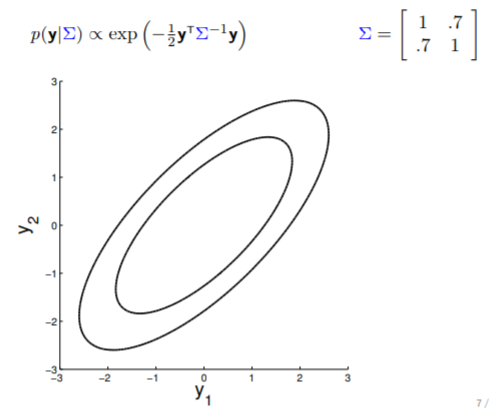
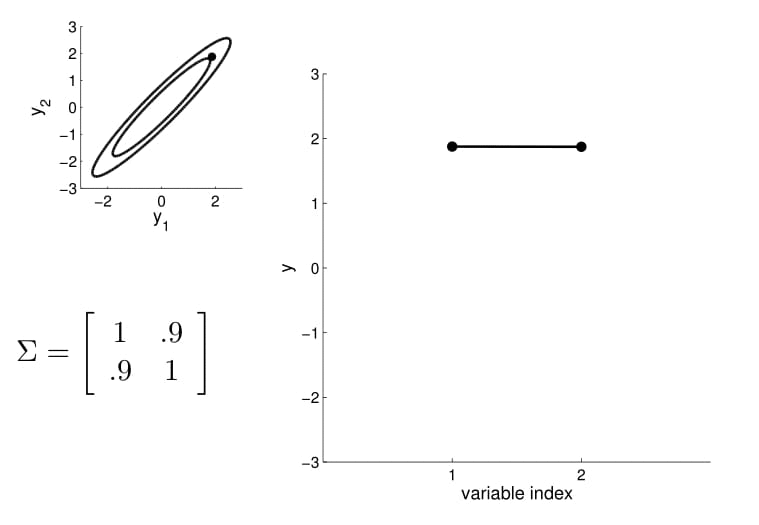
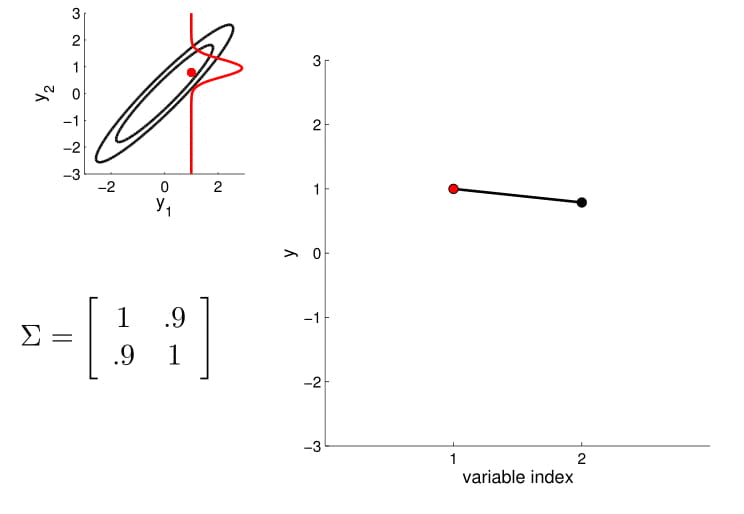
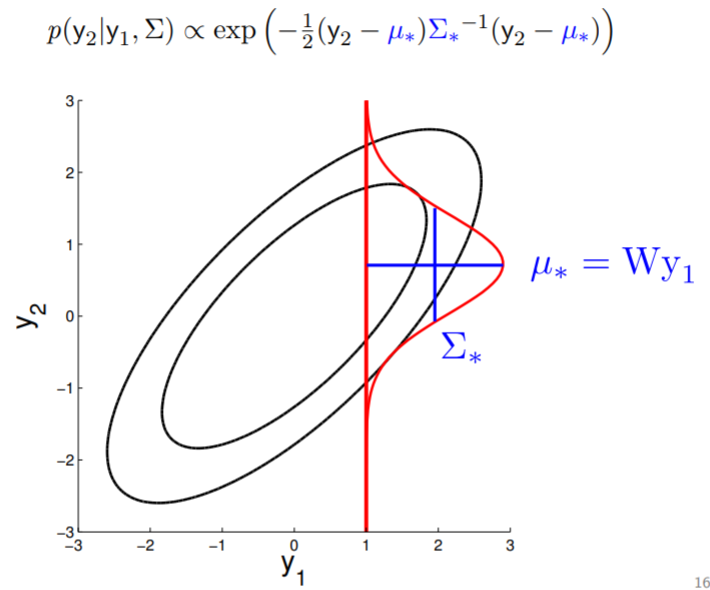
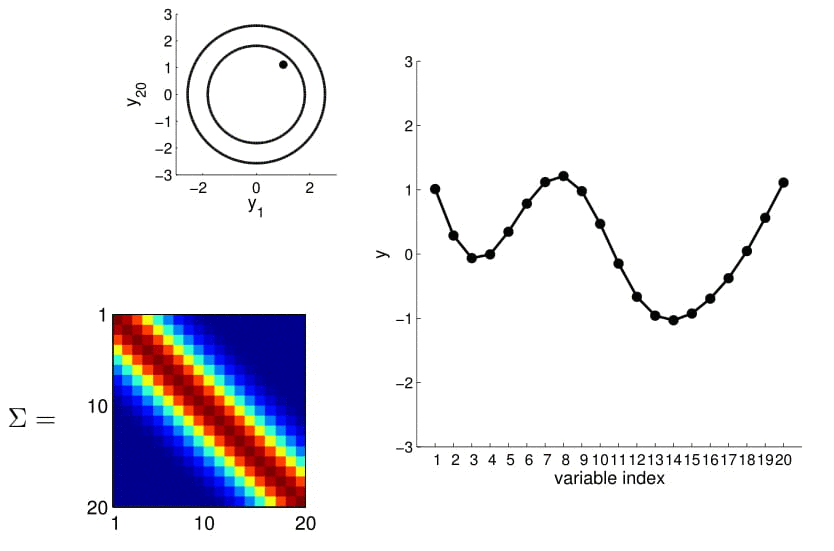
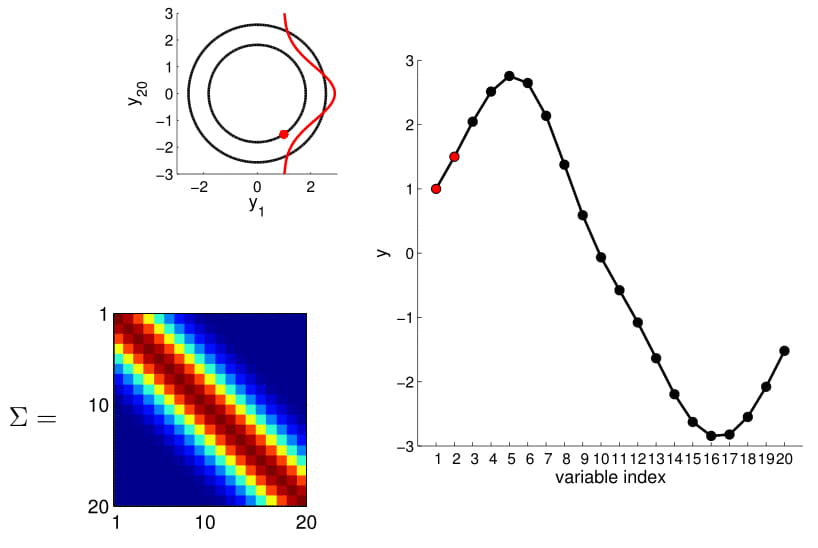
Conditional probability is,
\[ \begin{align} P(A|B) = \frac{P(A \cap B)}{P(B)} \end{align} \tag{1} \]
Get real number
It feels like all the points on the x-axis have to be integers because they are indices, while in reality, we want to model observations with real values.
We can calculate this covariance matrix for any real-valued \(x_1\) and \(x_2\) by simply plugging them in. The real-valued x's effectively result in an infinite-dimensional Gaussian defined by the covariance matrix.